For the past two decades, analysts have busied themselves planting acres of dashboards, reports, and visualizations, turning business customers into hunter-gatherers searching for morsels of insight hidden in picklists, columns, and graphs. But hunting & gathering is a labor intensive process, dependent on time, skill, and a good deal of luck.
Consider a key insight needed to inform an unsolved business problem. A leader searches for that undiscovered insight – that ‘needle in the haystack’ – among the thousands or millions of reports and filter combinations is a lot like trying to find a needle in the rows and rows of a large farm. With not enough time to search everywhere, the leader will have to either give up, or settle for the closest thing they can find to a relevant insight.
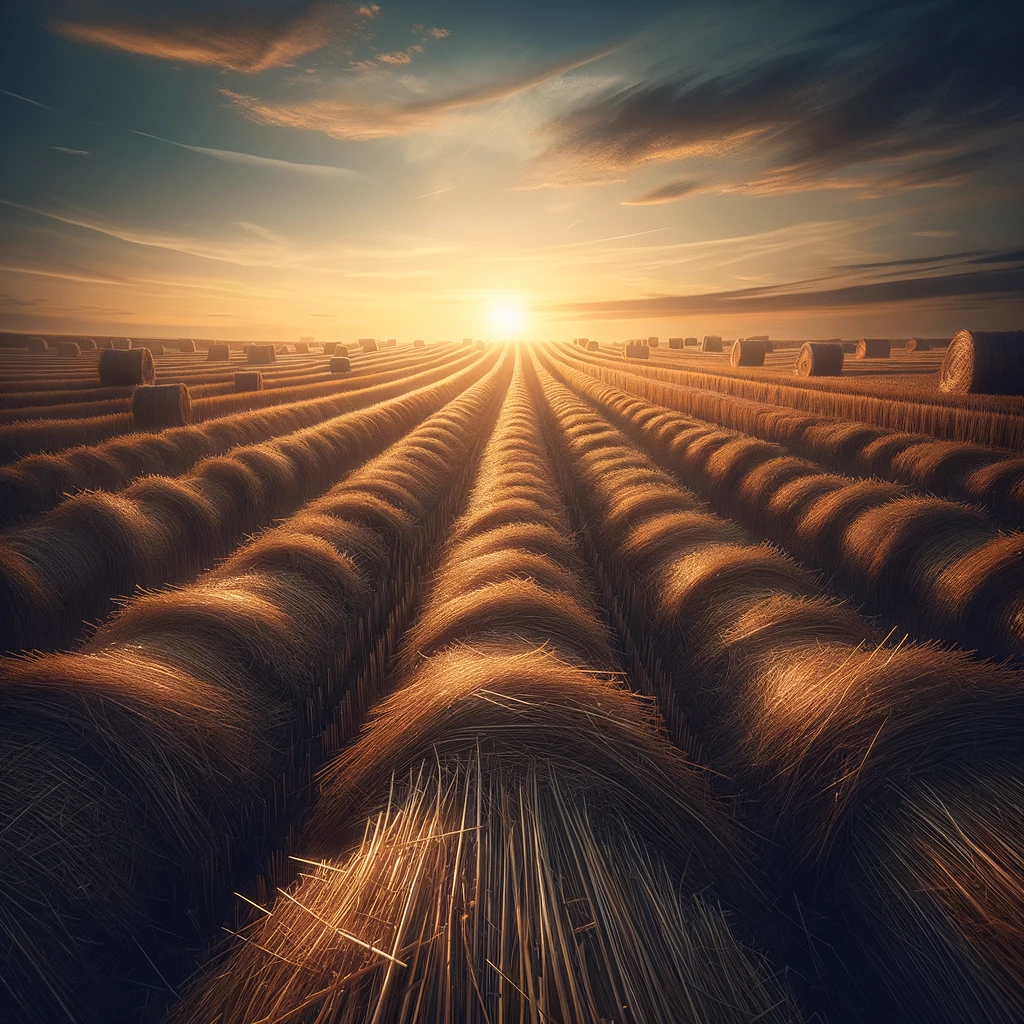
Even if the rows of hay were rolled into neat haystacks (or reports rolled into dashboards) finding the needle is still daunting. In analytics, we move from reports to dashboards and turn the leader lose to go search for that magic intersection of parameters where a needle or insight might be. Dashboards beat reports, but there’s still a lot of manual searching required to figure out where the needle is.
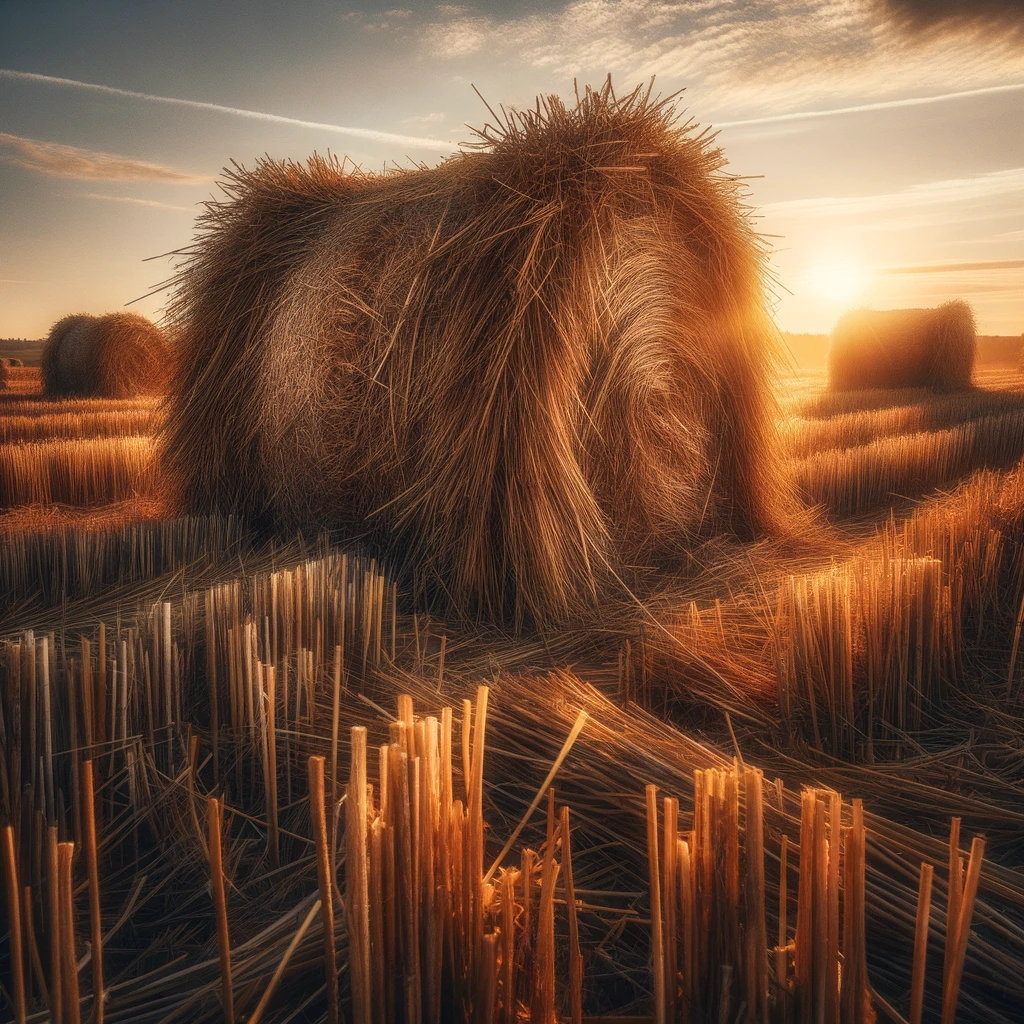
So what’s next, beyond reports, and beyond dashboards? The Age of Augmented Analytics, where artificial intelligence automates the endless searching and filtering. The analytics are augmented by machine learning to find statistically relevant insights, then explained in common terminology through natural language processing, and the leader is given those items that look like needles, or like insights. The leader must still execute their judgment to determine which needle they need, but the generated insights are available and delivered directly to the leader.
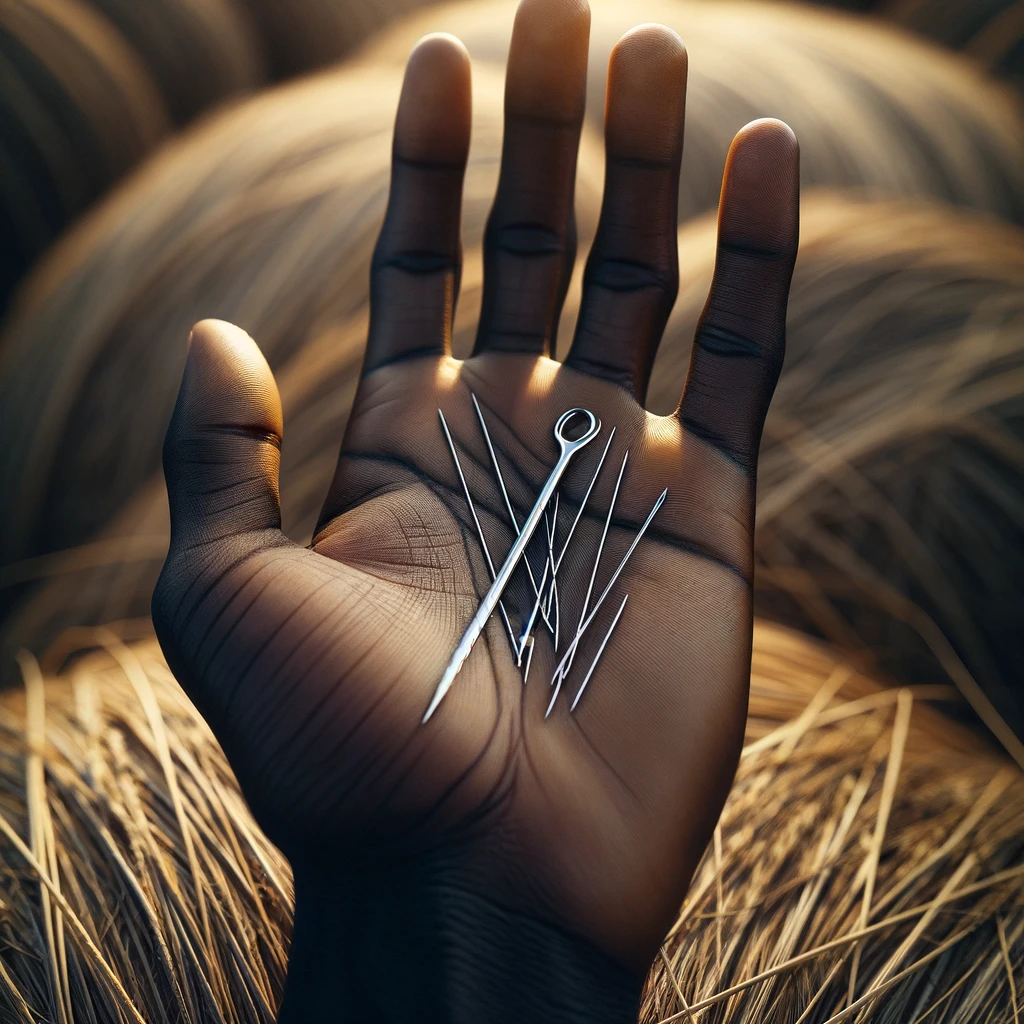
. Now, the leader can lead. And convert the searching time into decision and action time.
Augmented Analytics solutions are being increasingly built into the large computer systems leaders are already using for their work. Workday’s “People Analytics” is one such example. (Companies are adding this capability to other systems as well.) Scheduled to run as desired (e.g. monthly, after payroll runs, etc), People Analytics uses machine learning to evaluate every possible intersection of data to identify any statistically significant trends or gaps. Then, using natural language processing, the possible insights are surfaced to the leader in “plain English” terms they can understand.
The leader then evaluates each possible insight, using their additional real world context and business knowledge to determine which ones are relevant. Because the machine learning only knows the world from the data set it possesses, it will find ‘false positives’, or things that it thinks look like needles but aren’t. The leader’s additional knowledge of the business context outside of the data will be needed to make the final selection.
Augmenting analytics in this way saves leaders significant amounts of time, which can be spent acting on insights rather than searching for them. Goodbye, age of visual data discovery.